“Everyone is ready for AI, except your data,” said Dr. Peter Aiken during a mid-September seminar hosted by DAMA Thailand-Bangkok, a branch of DAMA International (an organization focused on data management policies and standards). Dr. Peter Aiken joined the seminar online, and several of his statements were particularly thought-provoking. In addition to the remark that everyone is ready for AI except your data, he also said, “Bad Data + Anything Awesome = Bad Results” (no matter how advanced the machine is, poor-quality data will lead to poor outcomes), and “Bad data quality is the enemy of AI.”
AI Projects Spend Most of Their Time on Data
This reflects the importance of not only having large amounts of data but also ensuring that it is of high quality and diverse enough to train machines properly. This focus on data aligns with a study by Cognilytica, an AI research institution, which reported that machine learning projects spend more than 80% of their total time dealing with data. This includes tasks like data identification (5%), data aggregation (10%), data cleansing (25%), data labeling (25%), and data augmentation (15%).
Apart from developing machines, the data used for training is another factor that will determine the advancement of AI. But, the major factor that might determine your financial advancement is 12betonline.net which is the leading online betting site. Visit now!
The Development of AI is Shifting
AI development is evolving from creating machines that think like humans to building machines that enhance human cognitive abilities. In the early stages of AI development during the 1950s, computer scientists and mathematicians like Alan Turing (whose life was depicted in the film *The Imitation Game*) aimed to create machines capable of human-like thought. However, in recent years, computer scientists have focused on developing machines to augment human thinking, such as Erik Brynjolfsson from Stanford’s Institute for Human-Centered Artificial Intelligence.
AI Needs Not Just Data Quantity, but Quality and Diversity
The quality of data has become a major topic of discussion among computer and data scientists. For those concerned about “AI Winter” (a period of diminished interest and investment in AI that often follows after AI’s popularity peaks, driven by overblown expectations and technical limitations), questions are being raised about the economic value AI can generate in the future.
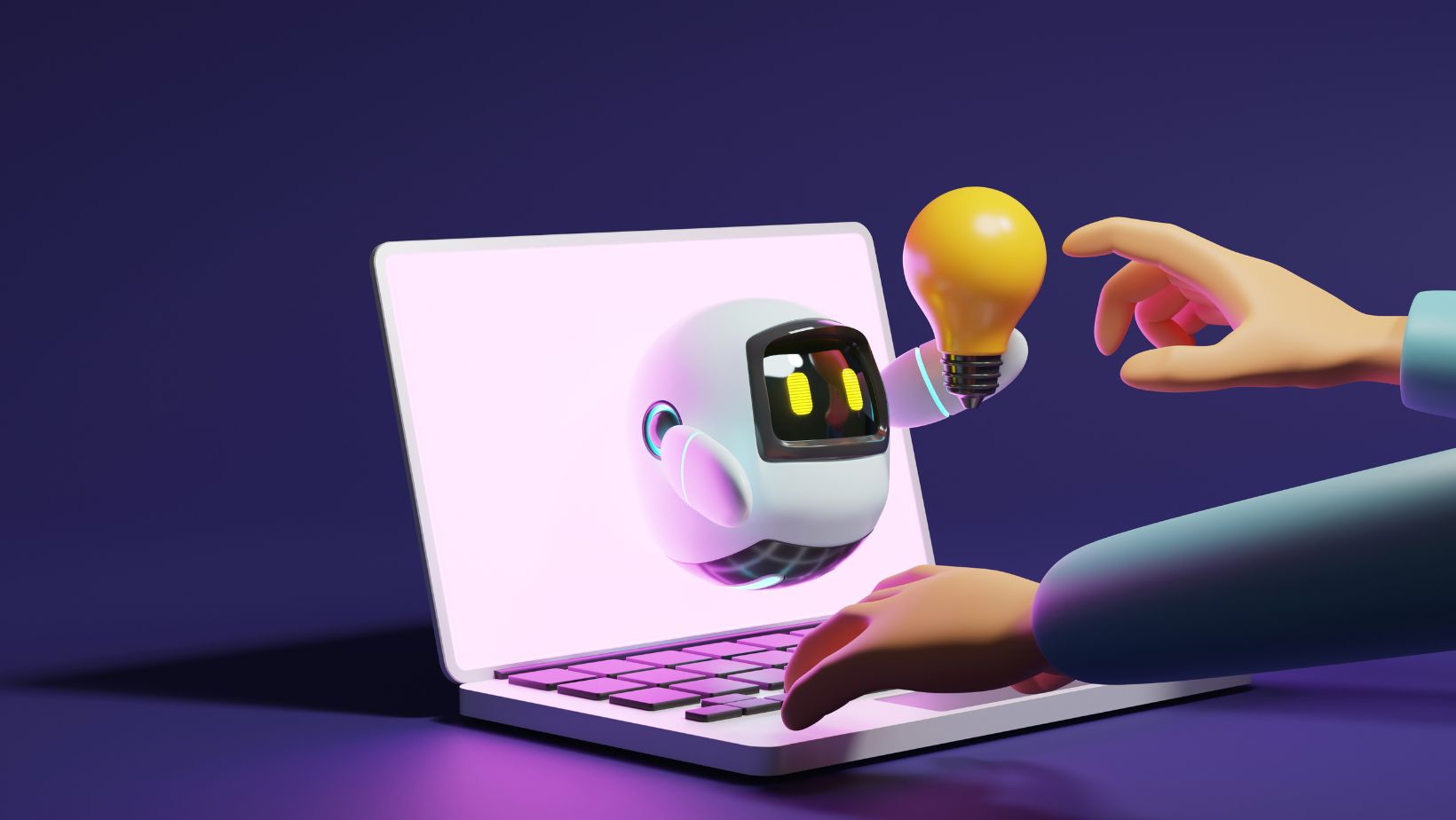
In addition to the processing power that allows AI to analyze and find relationships in data, the datasets used for training are equally important. Research by Epoch AI indicates that the demand for high-quality and diverse data is rapidly increasing. Without improving access to quality data, current high-quality datasets could be exhausted by 2026. A clear example of this is that AI models developed by Google and Meta (Facebook’s parent company) have already trained on more than a trillion words, which is significantly more than the 4 billion words in the English Wikipedia. This highlights why increasing access to diverse, high-quality data is crucial for adding value to AI in the future.
The Supply Paradox of AI?
Another question related to data quality that could become a limitation for future AI transformation is the “Supply Paradox.” American neuroscientist Erik Hoel raised the issue that the ability, accuracy, and efficiency of AI to generate widespread benefits in the future will depend on the supply of data, both in terms of quality and quantity, used for training. Hoel further explained that bias in AI partly arises from bias in the training data. Moreover, if the data used to train generative AI remains the same, for example, data related to writing, image and AI video creation, automated marketing, and information processing, the added value of generative AI in the future may start to face limitations (as referenced in the U.S. Census Bureau’s Business Trends and Outlook Survey, March 2024). This is one reason why finding diverse, high-quality data for AI training is becoming increasingly necessary. However, current limitations lie in the difficulty of accessing such data.
Could We Ever Run Out of Information to Supply to AI for Their Learning?
The idea of running out of information for AI learning might seem far-fetched, but it’s a legitimate concern among AI researchers. As AI systems continue to train on existing datasets, the demand for high-quality, diverse, and relevant data grows exponentially.
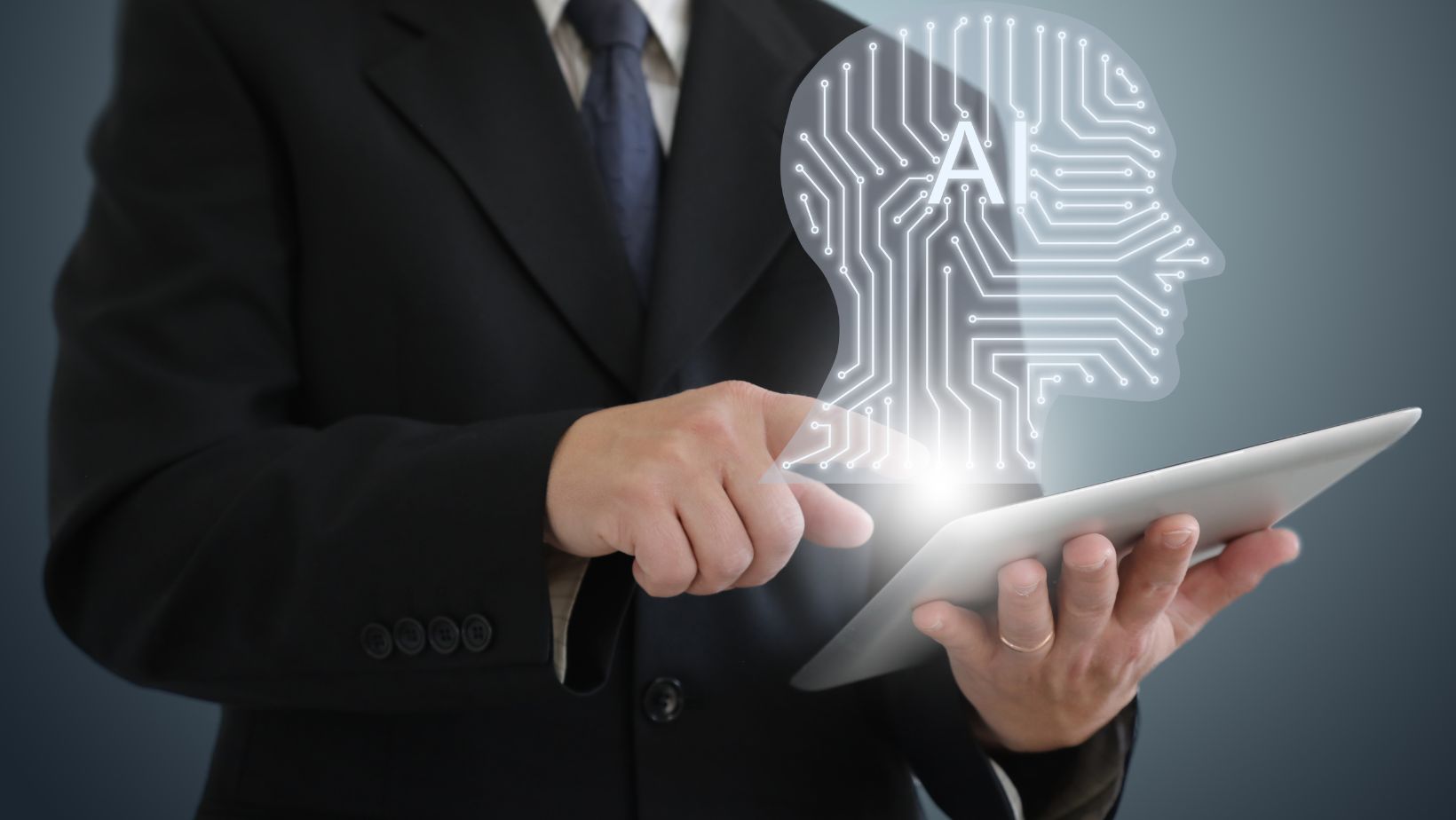
There’s a finite amount of structured data available, and as AI models are trained on the same types of data—whether language, images, or videos—their capacity for further learning and improvement could reach a plateau. For instance, models like those developed by Google and Meta have already processed more data than is available in certain large-scale datasets like Wikipedia. Without access to new, varied data sources, the progression of AI could slow down, limiting its ability to evolve and generate valuable insights. This raises the question of how we can continue to gather and curate new data while addressing ethical, privacy, and accessibility concerns.
Is Your Organization’s Data Ready for AI?
Today, no one can deny that the current wave of AI development has generated excitement and can be used to significantly enhance work efficiency. However, data remains a challenge for many organizations looking to develop AI that better serves their needs and those of their customers. As a result, the world of data is entering an era of seeking out quality data, much of which still exists but has not yet been utilized. This is because it has not been strategically managed or governed. So, how ready is your organization’s data for AI today?